Columbia Engineering researchers develop a novel approach that can detect AI-generated content without needing access to the AI's architecture, algorithms, or training data–a first in the field.
When ChatGPT was released to the world more than a year ago, many industries –and individuals–were very excited, and very fearful. Much of the fear centered around whether or not people’s jobs were going to be eliminated. And many were anxious about the rising potential of misinformation. In the education space, people worried whether students would have easier access to cheating on assignments and plagiarizing work.
Questions remain around how to enable students and teachers to adapt, rather than reject, ChatGPT and similar generative AI tools. At this point, is it even an option to opt out?
The answer is no, according to Vishal Misra, who is vice dean of computing and artificial intelligence at Columbia Engineering, a new position created by Dean Shih-Fu Chang. Misra, a professor of computer science and member of the Data Science Institute, has been tasked with leading the School’s initiatives in computing and AI and focusing on integrating these tools into our teaching and research activities while ensuring their ethical and responsible use.
Misra co-founded the popular website CricInfo, which was acquired by ESPN in 2007, and in 2021 he developed one of the world’s first commercial applications built on top of GPT3 for ESPNCricinfo. Over the past year, he has been modeling the behavior of large language models, reversing the traditional path from research to entrepreneurship. He also played an active part in the Net Neutrality regulation process in India, where his definition of Net Neutrality was adopted both by the citizen's movement as well as the regulators.
We wanted to learn more about his vision for this new position and how he sees AI impacting Columbia University. Here’s what he told us.
As the Engineering School’s first Vice Dean of Computing and Artificial Intelligence, how do you plan to integrate AI and computing tools into teaching and research activities while ensuring their ethical and responsible use within Columbia Engineering and the broader University community?
ChatGPT took the world by storm. AI has been making steady progress over the years, things accelerated with the advent of deep learning around 2011, but ChatGPT was the first time everyone could directly use AI - from a middle school student to a lawyer to a retiree. You don’t have to be tech-savvy to use cutting-edge AI anymore. But just because you can use it doesn’t mean you know how or when to use it. So one of my mandates is to ensure that the broader University learns to use AI ethically and responsibly, whether it is in teaching or research. This will be done via a variety of initiatives I hope to drive, from informal educational sessions to actual policy documents.
What advice and guidance were you already giving your students about the use of ChatGPT and similar tools?
My favorite analogy for ChatGPT since it came out has been to think of it as the most helpful and knowledgeable entity you can hope to encounter, but with early onset dementia! You really need to verify what it confidently asserts - hallucinations (incorrect, misleading information generated by AI) are a big problem. It is a great tool if used properly with a clear understanding of the limitations.
Given your extensive background in mathematical modeling and systems analysis, how do you plan to leverage your experience to bridge the gap between theory and practice in the development and implementation of computing and AI initiatives at Columbia Engineering?
I backed into doing research on LLMs (Large Language Models) like ChatGPT. The typical path that academics like me take is that they do fundamental research on some topic, and then down the line, they see some commercial application of their research and try to commercialize it. In my case, I first built a commercial application using GPT3 which has been running in production on ESPNCricinfo (a startup I co-founded when I was a grad student) since September 2021 - about 15 months before ChatGPT was released. In building the application and playing with GPT3, I became fascinated with how it works and started trying to build a mathematical model for it.
Now, together with my collaborator Professor Sid Dalal in the Statistics Department, we think we have a model that mathematically explains how and why these LLMs work, what their strengths and limitations are, and how they can help with research. I hope my experience both in developing mathematical models as well as building production applications using AI will help in providing the right kind of compute infrastructure so that Columbia Engineering and the University in general can leverage AI to the fullest.
Could you share some insights into your vision for strategic partnerships with other schools, institutes, and stakeholders both within and outside the University? How do you foresee these collaborations advancing Columbia Engineering’s role in computing and AI?
We have a great collaboration– the initiative of Empire AI– between the State of New York and six different universities. The legwork for that was done by our Executive Vice President for Research Jeannette Wing and Columbia Engineering Dean Shih-Fu Chang. I hope to help them make that vision become reality and ensure that Columbia’s research community has similar compute capabilities available like the big tech companies (Google, Microsoft, Amazon, etc.) or companies like Open AI. We are also in active discussions with major cloud providers to build strategic partnerships so that we can leverage their compute infrastructure in a mutually beneficial way -- they give us access to their computer resources, we develop new applications and uses via our research, and in turn, their compute resources get used more.
As far as other stakeholders within the University, all this is very much in play. AI has hit all of us right in the face, but the computer science department and the Engineering School are the natural leaders in making sure that everyone in our community is using these tools in the right way, maximizing productivity while using them ethically and thoughtfully.
Do you currently have a go-to or favorite chatbot tool?
ChatGPT4 is still my current favorite tool, but I have recently started playing with Gemini and it is very promising. Things are changing by the minute in this world!
How are your students currently using these AI systems?
I have a PhD student who is actively doing research using these AI systems, and also a few undergrad students working on them as well. My other students use them to essentially do data wrangling (and help with some paper editing).
Will you still have time to play cricket?
Let’s see when summer comes - hopefully yes! This year the T20 cricket World Cup is being hosted in the Caribbean and the U.S., and New York has some marquee games like India vs. Pakistan. So I hope to not only play but also watch some top-flight cricket this summer.
Is there anything else you would like to say?
Stay tuned. A lot of exciting things are going to happen!
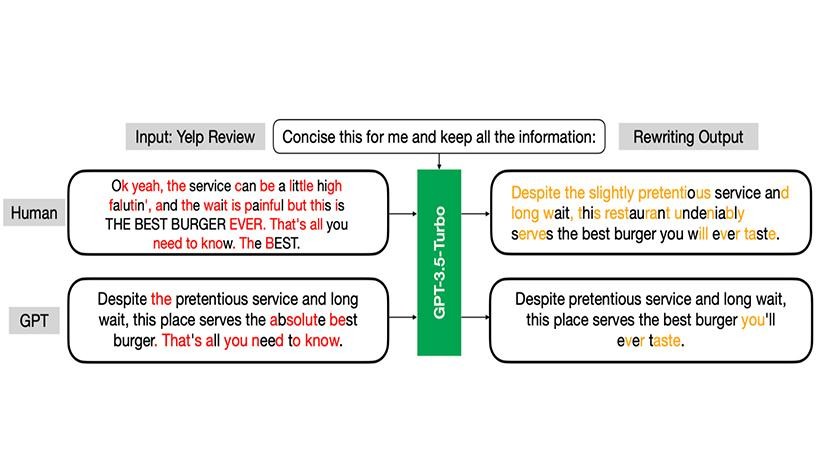
Raidar's remarkable accuracy is noteworthy -- it surpasses previous methods by up to 29%. This leap in performance is achieved using state-of-the-art LLMs to rewrite the input, without needing access to the AI's architecture, algorithms, or training data—a first in the field of AI-generated text detection.
Raidar is also highly accurate even on short texts or snippets. This is a significant breakthrough as prior techniques have required long texts to have good accuracy. Discerning accuracy and detecting misinformation is especially crucial in today's online environment, where brief messages, such as social media posts or internet comments, play a pivotal role in information dissemination and can have a profound impact on public opinion and discourse.
Authenticating digital content
In an era where AI's capabilities continue to expand, the ability to distinguish between human and machine-generated content is critical for upholding integrity and trust across digital platforms. From social media to news articles, academic essays to online reviews, Raidar promises to be a powerful tool in combating the spread of misinformation and ensuring the credibility of digital information.
"Our method's ability to accurately detect AI-generated content fills a crucial gap in current technology," said the paper’s lead author Chengzhi Mao, who is a former PhD student at Columbia Engineering and current postdoc of Yang and Vondrick. "It's not just exciting; it's essential for anyone who values the integrity of digital content and the societal implications of AI's expanding capabilities."
What’s next
The team plans to broaden its investigation to encompass various text domains, including multilingual content and various programming languages. They are also exploring the detection of machine-generated images, videos, and audio, aiming to develop comprehensive tools for identifying AI-generated content across multiple media types.
The team is working with Columbia Technology Ventures and has filed a provisional patent application.